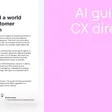
How to build a world-class AI customer service team
Templates and guidance on building a customer service team that uses both AI and human agents to their fullest potential.
Learn MoreAI agents aren’t plug-and-play solutions. Think about it: You wouldn’t hire a new support agent and expect them to operate at full capacity without training, right? Just like human employees, you need to coach AI continuously to reach peak performance.
That’s where AI coaching comes in—the process of refining your AI’s ability to handle more complex inquiries, personalize interactions, and align with your customer service goals.
After years of working as an AI manager and supporting AI managers at other companies, I can confidently say: the best AI agents aren’t the ones with the most data—they’re the ones that are coached on how to best use it. A well-coached AI agent is so much more than your run of the mill “chatbot”—it’s a digital team member that learns, adapts, and improves over time.
But where do you start? If you want an AI agent that delivers seamless, human-like customer experiences—handling complex inquiries, resolving issues accurately, and adapting in real time—you need to approach coaching with intention. Here’s how to set your AI agent up for success.
Your AI is only as good as the data it learns from. That’s why your AI agent’s first teacher is your knowledge base —but not all knowledge bases are AI-friendly.
In my past role as an AI manager, I found that there are types of content that work well for human consumption that don’t work for AI. While a human can easily scan a table or an infographic for relevant data points, it’s difficult for AI to retrieve the right answers.
But don’t go scrapping the content altogether— there’s a better solution . Here’s how you can reformat your existing information in a way that AI can understand:
If you do this, you will see immediate improvements in response accuracy. Training your knowledge base to be AI-friendly is the foundation of effective coaching.
By optimizing your knowledge base, you’ll not only boost your Automated Resolution rate but also maximize cost savings, improve efficiency, and deliver better customer experiences.
Get the guideEven with a solid knowledge base, your AI agent won’t get every response right the first time. And constantly updating the knowledge base to correct minor AI mistakes isn’t scalable.
Instead, use AI coaching tools to refine responses at the conversation level. This ensures that the AI understands context, learns from past mistakes, and improves dynamically. Instead of rewriting your entire knowledge base every time something isn’t working, try the below:
It’s easy to get caught up in fixing individual misfires, but AI coaching is most effective when it’s guided by patterns.
Instead of trying to "fix" AI every time it makes a mistake, start setting coaching rules that adjust the AI’s response based on real interactions. This way, you can employ precise, targeted improvements across a broader range of conversations—and ensure that corrections apply only where needed.
A great AI agent doesn’t just provide accurate answers—it provides personalized ones.
Accuracy alone isn’t enough. Customers don’t want to interact with AI that is blatantly robotic and impersonal, and generic AI responses often lead to frustrated customers and escalations.
To create a human-like, AI customer experience, coach AI agents to be able to pull in customer-specific context, like purchase history, past interactions, and customer sentiment. To do this, AI managers should:
By embedding customer data into AI conversations, businesses can create more meaningful, relevant interactions—reducing escalations and improving satisfaction. For example, if a repeat customer reaches out about a delayed order, the AI should recognize them, acknowledge their previous purchase, and provide an update specific to their order rather than delivering a generic shipping policy.
Don’t let your AI operate in a vacuum. Connect it to customer data so it can offer relevant, contextual responses.
One of the biggest mistakes an AI manager can make is to only focus on the most common inquiries. It’s tempting to assume that if AI gets the top 10 FAQs right, then it’s working well. But not all AI failures are obvious.
In reality, AI failures tend to happen in edge cases, not the easy stuff. Testing beyond top inquiries is crucial—some of the biggest AI failures happen in complicated workflows, not the most frequent tickets.
AI managers should take a proactive approach to coach AI by identifying weak spots and course-correcting before issues escalate by:
The only way to catch these failures is to test for them intentionally. Instead of only evaluating how AI handles frequent, simple questions, AI managers should proactively test it with multi-step, complex inquiries.
Looking at AI analytics reports can also reveal hidden issues—if certain inquiries are being escalated at a high rate, that’s a sign the AI needs additional coaching in that area.
AI agents aren’t static scripts—they are designed to learn and adapt. One of the biggest mistakes AI managers make is overcoaching—constantly tweaking AI responses to "perfect" every interaction.
When too many coaching rules are applied, they can contradict each other, creating unpredictable results—aka, making the AI’s behavior worse.
The goal is to coach AI strategically, not reactively, with a clear strategy, focused on the right areas, and given room to adapt within reasonable guardrails. Here’s how you find the right balance:
AI coaching should be targeted and intentional, ensuring steady improvements without micromanaging the AI’s learning process. Ask yourself: Is this a high-risk topic that requires strict control, or is it okay for AI to adapt naturally?
A well-coached AI agent doesn’t just provide answers—it understands context, adapts to customer needs, and continuously improves. AI managers who take a structured, data-driven approach to coaching will see:
Again, coaching isn’t about fixing every mistake—it’s about guiding AI toward better decision-making over time. A well-coached AI agent doesn’t just answer questions—it understands, adapts, and improves. This means coaching with intention, monitoring consistently, and refining strategically.
AI coaching is the secret to AI-driven customer experiences that feel human. Start coaching today, and watch your AI agent evolve.
A strong knowledge base is the difference between AI that confidently resolves customer inquiries and AI that fumbles, frustrates, and escalates. Learn how to turn your knowledge base into AI’s greatest asset.
Watch webinar