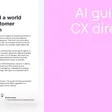
How to build a world-class AI customer service team
Templates and guidance on building a customer service team that uses both AI and human agents to their fullest potential.
Learn MoreIf you’ve got your finger on the pulse of AI, you’ve no doubt heard of “hallucinations.”
While you're more likely to see us highlight the benefits of generative AI for customer service on any given day, we also recognize the importance of addressing the challenges that come with cutting-edge technology. Especially when 86% of online users have encountered an AI hallucination firsthand.
But AI hallucinations don’t have to derail your customer service. As AI-powered solutions become increasingly integrated into customer service operations, understanding this phenomenon is the first step to ensuring accurate and trustworthy automation experiences.
Embracing a proactive and holistic approach to preventing, detecting, and correcting hallucinations are your next steps, and in doing this, businesses can unlock the full potential of AI while maintaining the highest standards of accuracy and reliability.
Here’s everything you need to know about AI hallucinations to get there: what they are, their impact on your customer service, and the strategies for detection and prevention.
AI hallucinations occur when an AI model generates outputs that are plausible but factually incorrect or inconsistent with the given context. These hallucinations can manifest in various forms, such as:
In the same way human error can be the result of a variety of factors, AI is susceptible to hallucinations for an array of reasons, including (but not limited to) the below:
Prompt formulation: The way prompts or queries are formulated can influence the AI model's response, and ambiguous or poorly constructed prompts can increase the likelihood of hallucinations.
Hallucinations can occur in up top 28% of responses generative by large language models.
- Anthropic
In the context of customer service, AI hallucinations can have far-reaching consequences. 62% of customers say they will stop doing business with a company after just one or two negative experiences, so trust us when we say hallucinations are something you want to avoid at all costs.
More specifically, AI hallucinations in customer service can lead to:
First, let’s address the most commonly asked question we get as an AI-powered automation platform for customer service: “Is it possible to create an AI agent that never hallucinates?”
The short answer is no. But we can get close. There are ways to mitigate and minimize hallucinations, but current AI technology isn’t perfect and can’t ensure this will never happen.
Think about it this way: you can’t ensure that your human agents — trained experts in your product, services, and brand — won’t make mistakes in their jobs from time-to-time. 66% of agents in call centers reported the primary reason they make mistakes on a call is human error. The reason we’re not seeing this covered as much as AI hallucinations, is that AI has a lower acceptable error rate than humans.
"Preventing AI hallucinations requires a multi-faceted approach that combines advanced techniques like RAG with rigorous data curation and continuous model improvement.”
- Dario Amodei, CEO of Anthropic
But what exactly does a multi-faceted approach to preventing AI hallucinations look like? Here are some ways you can mitigate AI hallucinations:
Still, even with preventative measures in place, AI hallucinations can occur. Researchers found that AI hallucinations may happen as infrequently as 3% or as often as 27% depending on the AI model you’re using (and how you’re using it).
If your AI agent has measurement and analysis tools in place, it’s easy to identify hallucinations and quickly correct them. For example, Ada’s AI Agent has Automated Resolution Insights that highlight opportunities to fill in the gaps in the knowledge base or create new actions to improve your Automated Resolution Rate .
Here are some strategies for detecting and correcting AI hallucinations:
"Effective human-AI collaboration is crucial for mitigating the risks associated with AI systems, including hallucinations.”
- AI Now Institute
AI hallucinations aren’t an insurmountable obstacle, but rather an opportunity to continuously refine and enhance our AI systems. While AI hallucinations still present a challenge, these strategies can deter and minimize the potential occurrences.
Despite this risk, our AI Agent is still automatically resolving 70% of customer inquiries. By continuously improving our training data, models, and knowledge bases, implementing advanced techniques like RAG, and fostering human-AI collaboration, we’re able to minimize the occurrence of hallucinations and ensure the accuracy and reliability of our AI Agent.
With the ability to learn and grow over time to reduce the risk of hallucinations, much like an employee, an AI agent can go above and beyond expectations.
Discover the key factors that contribute to hallucinations, learn best practices for prevention, and get actionable tips on how to quickly identify and correct them.
Get the guide