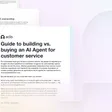
Guide to building vs. buying an AI Agent for customer service
This guide will help you assess the build vs buy question from 5 main angles so you can make a confident, informed decision.
Learn MoreAI is coming for your customer service workflow.
This isn’t a case of AI coming to life and taking over the world (like some doomsayers are hand-waving about). Rather, business leaders in need of solutions for customer service management are finding what they need in AI — and they’re jumping at the chance to transform their processes in its image.
And it's clear why. In a recent survey of customer service professionals conducted by Hubspot, 78% said that AI/automation helps them spend more time on the more important parts of their roles. Additionally, the authors of a recent Forrester report examining the state of CCaaS (Contact Center as a Service), declared that “AI was not really a consideration; it now permeates every element of a differentiated CCaaS offering, from self-service to post-contact analytics.”
Already, AI is being used to speed up the creation of support content, automatically generate answers without support agents having to manually build them, and better analyze and optimize support conversations, which can improve how feedback is routed (and ensure it doesn’t get lost). And there are a lot more benefits and efficiencies AI can unlock for customer service in the future.
"78% of customer service professionals say that AI/automation helps them spend more time on the more important parts of their roles."
- HubSpot
But AI isn’t a cure-all for the industry. AI can’t do customer service entirely on its own, and human customer service workers have to build new systems and overcome challenges to reap its benefits.
Here’s a look at what AI can’t do for customer service and where humans will be especially important in coaching the next generation of customer service workflows.
One misconception is that the AI-powered chatbot companies are increasingly deploying to interact with customers will be the be-all and end-all of customer service. But the idea that, because AI may be on the frontlines it will be an exclusively AI-process through and through, isn’t the full picture. Humans will not only remain in the loop to create these chatbots and other AI-powered customer service tools, but they’ll play crucial roles in managing them, setting them up for success, and coaching them to get better over time.
“Agentless is not humanless.
It’s important to note that while automation will eventually supplant human agents almost entirely, human customer service agents will be elevated to a more strategic and coaching role in the company.”
This is changing how clients structure their customer service organizations and create new career paths, he said. Customer service leaders should keep an eye on what skill sets they already have on the team and where they can fill gaps as AI creates new ways human customer service professionals are needed.
For example, take generative AI . Companies can use this most recent and fast-moving branch of AI to automate their most common FAQs, handle complex contextual conversations, answer follow up questions and engage in chit chat by integrating across systems, but human workers still need to be involved through various steps. This includes, creating and maintaining the brand persona for the generative AI-powered customer experience, integrating the technology across various systems, and continually optimizing it using analytics.
“The AI is there to just speed up the process, but at the end there's a human to review that what has been generated is actually relevant, accurate, and safe,” said Mike Gozzo, Chief Product and Technology Officer at Ada. And in the near future, humans may not even have to review all generated actions and instead will be able to focus on coaching the AI to learn faster and more efficiently.
But for now, the need for humans to review the content is especially true when it comes to using off-the-shelf models, which need to be tailored to each company’s needs and require extra scrutiny to safeguard against unhelpful and even harmful interactions.
“If you’re incorporating a publicly available large language model (LLM) into your techstack, you need to make sure you have a deep understanding of how this technology works and what kind of guardrails you’d need to set up around it to make sure you don’t end up on one of those AI generated fails Twitter accounts. What you can't do essentially is just deploy it, set it, and forget it, without putting some mechanism or process in place to validate that the generated output is safe, accurate, and helpful.”
Just as AI won’t be able to do everything on its own, it won’t be able to do everything (yet).
AI is excellent at combing through and making sense of massive amounts of data. It’s great at recognizing patterns. And now with the latest breakthroughs in generative AI, it’s become increasingly conversational. But AI is still limited to the domains on which it’s trained.
“One thing to remember is that AI is limited by the information we feed it,” Béné Pierrejean, senior machine learning scientist at Ada, said. “While it can combine and synthesize information from multiple sources to help customers solve their problems, it cannot come up with ad hoc solutions that may be specific to a business.”
For example, imagine you're a customer trying to get answers about your missing package.
“While a support agent could help you get in contact with the shipping company and locate the package, it is currently not possible for an AI to do that all on its own,” said Pierrejean.
Similarly, an AI-powered chatbot trained to handle a specific type of customer issue won’t automatically be successful if the company opens up a new line of business that’s prone to a whole different set of customer inquiries. Additional training could help fill the gap, but AI systems aren’t equipped to make such a jump on their own.
Artificial general intelligence (AGI), a hypothetical form of AI that could examine scenarios and determine actions outside the bounds of what it was taught, could change this. But researchers continue to debate how long until AGI is finally realized — and if it’s even possible at all.
As mentioned, companies using AI for customer service can’t set it and forget it . AI — and the advanced insights it makes possible for businesses to uncover — can bring intelligence to customer service workflows, but it certainly can’t make them error proof. In fact, while artificial intelligence opens up a host of new possibilities and efficiencies, it also introduces new avenues for error.
For companies using publicly available LLMs to power customer-facing chatbots and other AI-powered customer service technologies, the issues surrounding training data are paramount and have real effects. The datasets used to train these models — typically content scraped from the internet — were incomplete, plagued with bias, and have been shown to perpetuate discrimination. Researchers and the technologists who developed these models have proven this many times over, and it’s even become clear through dramatic public showings. Microsoft famously had to delete its AI Twitter chatbot experiment Tay after only 24 hours after the bot started spewing racist statements, which it picked up from users. That was years ago, but the same thing happened with Meta’s BlenderBot 3 just last year.
In a collaborative LinkedIn article exploring the benefits and challenges of using AI chatbots for customer service, professionals in the AI space brought up these issues with data and concerns about entrenching real-world bias and discrimination. A recent Forrester report about customer service in 2023 echoed this, warning against unpredictable answers and inherited bias.
“If you’re using a publicly available LLM to power AI in a customer-facing chatbot, the AI is at risk of serving answers that are irrelevant, inaccurate, or harmful. More than ever, subject matter expertise and reliable sources are necessary to make the most of generative AI,” Gozzo said, adding that it’s critical for companies to tap all available guardrails to combat this, including ensuring that the AI they’re using in customer service automation only distills information found in their support documentation.
Beyond data concerns, generative AI is facing roadblocks in terms of the “hallucination” problem, wherein AI systems make up information that may sound true, but isn’t. It stems from the way that generative AI works, using patterns to predict the next word in a sequence, and some researchers believe that the hallucinations problem may not even be fixable .
Generative AI’s penchant for making errors has clear consequences for customer service use cases, where giving useful and accurate information is the whole game.
It's even more important than ever, in the ‘Age of AI’ that we are currently entering, for brands to be hyper vigilant about what their chat AI is presenting to their clients. The risk of hallucinations, inaccurate messages, or unsafe responses is very high without the proper controls and governance in place to monitor and improve the AI.”
There’s a lot AI can do to simplify customer service workflows, from speeding up the creation of support content to making it possible to tend to more customers quickly. But introducing AI, a deeply complicated and fast-moving technology, into the picture creates some new needs for businesses as well.
For example, the use of AI requires an evolving tech stack, complete with data, analytics, cloud, and other tools. And of course, technical expertise and efficiency is required to make sure all of these work in concert and deliver on the value they can offer. Plus when any changes are introduced, it’s important to make sure they don’t cause any new issues or interrupt the greater system.
The aforementioned issues around data and hallucination, in addition to being at risk of introducing errors, also demand additional attention and processes.
The uncertainty brought by the generative nature of the models makes the needed evaluation processes a lot more difficult, said Pierrejean. And for this reason, it’s necessary to measure not only a model’s accuracy, but also its capacity to hallucinate.
“Before generative AI, a lot of answers were hand-crafted and as such, you would know exactly what will be presented to the customer,” said Pierrejean. “This has become a challenge with generative AI where there is a risk that the model hallucinates. As a consequence, you want your evaluation process to be very thorough.”
With the finer points of what AI can and can’t do, the guardrails needed to make it run smoothly, and the benefits it can deliver all laid out, it becomes easy to imagine how AI can be at the heart of every customer service resolution.
Even though it may need a little human coaching (and don’t we all), AI is primed to deliver more efficiency for organizations, more elevated roles for countless customer service professionals, and faster, better experiences for customers.
This is especially important for improving the overall experience, as the quality of a customer’s interactions with a company can quite literally make or break not only a sale, but their perception of the company forever going forward forever. Half of consumers will switch to a competitor after just one bad customer service experience, and 80% will switch to a competitor after more than one bad experience, according to a 2023 report by Zendesk.
AI presents a bold opportunity to truly deliver on the promise of customer service and thoroughly help every customer, every time they need it.
“Customer service ultimately has one objective: provide excellent service to the customer," Monroe said. “This will never change.”
Practical guides to evolve your team, strategy, and tech stack for an AI-first future.
Download toolkit