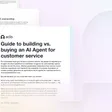
Guide to building vs. buying an AI Agent for customer service
This guide will help you assess the build vs buy question from 5 main angles so you can make a confident, informed decision.
Learn MoreIn a recent report on the modern AI stack , Menlo Ventures published a very telling statistic: almost 95% of AI spend is now on inference (or running AI models) rather than training them, according to the firm’s survey of more than 450 enterprise executives.
The number represents a massive sea change. Not long ago, a much wider set of companies were training AI models, and it was thought most companies would one day create their own unique AI models from scratch. Whether to “ build versus buy ” a model was a common contemplation among corporate IT teams exploring how to incorporate AI, and many set off building their own unique models for their own purposes.
“Teams seeking to build AI applications needed to start with the model — which often involved months of tedious data collection, feature engineering, and training runs, as well as a team of PhDs, before the system could be productionized as a customer-facing end product,” reads the Menlo Ventures report.
LLMs have flipped the script, shifting AI development to be ‘product-forward.
- Menlo Ventures report
In today’s AI landscape, companies like OpenAI, Anthropic, and Meta have done a lot of the heavy lifting, creating powerful large language models companies can tap as a starting point for their own products in customer service and beyond. But the widespread use of the same models has ignited a new approach to AI where it’s what a company does with a model once it's in hand that truly counts. Now that models themselves no longer serve as the main differentiator, companies are shifting their AI strategies to focus on supercharging leading models with their own data and processes.
The recent proliferation of highly capable LLMs available through APIs and open-source has made it easier than ever for companies to build AI-based products. Utilizing existing AI models is not only more efficient than creating a model from scratch, but far more cost-effective, too.
Today’s models are larger, faster, and require more cloud compute and specialized hardware. This has caused the costs of building models to skyrocket — and raised the barriers to creating models from scratch. According to the Stanford Institute for Human-Centered Artificial Intelligence (HAI)’s 2024 AI Index report , OpenAI’s GPT-4 cost an estimated $78 million to train, while Google’s Gemini Ultra cost an estimated $191 million.
The availability of models like GPT-4 allows companies across industries to build products using the most cutting edge AI available today. But the common use of the same LLMs has kicked off a new race in how companies in customer service and all industries can lead with AI.
Briana Browell, founder and CEO of Pure Strategy, has seen this first-hand. She’s spent the last decade working with companies of all sizes on their AI and data strategies to help them build better products, and over the last few years, she’s seen a massive change in how companies are approaching AI now that these models are available.
“I think that because the quality of the general models are so good right now, a lot of the way that we need to think about customization and sort of the use of the models is really different."
- Briana Browell, Founder & CEO, Pure Strategy
The trend is further demonstrated by the rise of retrieval-augmented generation (RAG) and prompt engineering — emerging techniques for working with LLMs that have taken the industry by storm over the past year or so.
RAG makes it possible to give a model information it was never trained on to reference and use in its responses. For this reason, it’s become one of the hottest topics in AI as companies look for ways to make general models work for their specific purposes. RAG is so useful because it allows companies to introduce their own proprietary data into a model, which is more important than ever in today’s AI landscape.
In the Menlo Ventures’ report , the authors break the modern AI stack into four layers. Layer one consists of the foundation models themselves, as well as the infrastructure required to train and ultimately deploy them. The next layer is all about the data.
Snowflake’s Head of AI Baris Gultekin and Accenture Global Senior Managing Director of Data & AI Tom Stuermer wrote in a blog post published last month:
“The greatest value from large-scale machine learning and generative AI will be realized when companies can rely on their own data to deliver the unique insights and recommendations that will fundamentally move the performance needle.”
They continued, “Then they’ll be able to go from interacting with a generic internet-trained chatbot to generating highly relevant content that leverages up-to-date and potentially confidential enterprise information.”
In fact, not incorporating timely and proprietary data is setting up a product to fall behind. The risk in basing your product on a general model is that once a better model comes out, you might have to rethink your product, says Browell. You can’t depend on the model to differentiate a product, so you need to think about the specific value the model can bring to your customer.
“Data is definitely one way to do that,” she says.
In the case of customer service technologies like AI agents , integrating data specific to the business is crucial. The information an AI agent has access to — its knowledge — dictates everything about how it understands customers’ inquiries, responds to them, and its ability to give them the information they need and solve their problems.
“It’s important to have high quality data for your AI agent because that’s going to inform how it responds. The quality of your systems will to some extent dictate the quality for your customers.”
- Gordon Gibson, Director of Applied Machine Learning, Ada
Ada suggests “onboarding” an AI agent because just like a human, it requires being set up with the information and processes it needs to perform the job well. This includes connecting the AI agent to a knowledge base so it has the most up-to-date and relevant information needed to help customers.
This is an important first step, but it doesn’t stop there. Guidance, also called feedback or instructions, is also critical for coaching AI agents on how to improve their performance and better resolve customer issues. This involves offering feedback on how it responded to a specific inquiry, or even giving it access to additional data it can use to respond better.
“Your AI agent will have some gaffes, or some areas that it’s not performing as well as you’d like it to. So having a platform that allows you to identify what those are very quickly and rectify or improve them through feedback and mechanisms is probably one of the most important aspects of a system.”
- Gordon Gibson
More than ever with today’s widespread use of general models, how a company integrates feedback will have a significant impact on its product, and thus the customer experience. And it all speaks to the larger point — data is the differentiator.
Customer service data has always been powerful, providing insights that can supercharge the entire business . Now in today’s new era of AI, data takes on an even larger role for customer service. Tapping proprietary data can transform common off-the-shelf models into highly specialized platforms designed to deliver on customers’ specific needs. It can empower the development team to seamlessly identify and make needed improvements. And it can make the difference between an AI agent that resolves customer issues and one that needs to escalate to a human like an old school chatbot.
Looking to better understand the difference between chatbots and AI Agents? Download this guide to become an expert on the topic, and discover the success criteria you should be testing to get the most ROI.
Get the guide