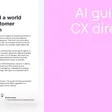
How to build a world-class AI customer service team
Templates and guidance on building a customer service team that uses both AI and human agents to their fullest potential.
Learn MoreChances are you’ve talked with a customer service chatbot recently. But did it actually solve your problem?
That’s the big question every company deploying chatbots for customer service is trying to answer. And as breakthroughs in AI transform customer service processes and unlock chatbots for use across new, more complex use cases, it’s becoming more important than ever.
This is in part because the use of AI-powered chatbots for customer service is increasingly taking center stage. Gartner last year projected that 10% of customer service agent interactions will be automated by 2026, a sharp increase from its estimate that 1.6% of interactions today are automated using AI. Many companies are also looking to advance chatbot technology to a level where it will be able to fully handle many customer issues without the need to escalate to a human , which makes measuring when a chatbot does so successfully paramount. The problem is, it’s becoming ever more clear that the industry’s go-to metric for measuring this, containment rate, is falling short.
“CS leaders have a positive future outlook for chatbots, but struggle to identify actionable metrics, minimizing their ability to drive chatbot evolution and expansion, and limiting their ROI."
Across the industry, customer service leaders are increasingly ringing the alarm about containment rate and working to establish new metrics for chatbot success. Here’s a look at why containment rate fails as an automation metric, and measures of success that can be tapped instead, such as automated resolutions (AR%).
Containment rate refers to the percentage of users who interact with a chatbot and then leave the conversation without speaking to a human agent. The idea is that if a user doesn’t escalate beyond the chatbot to get help from a human, the chatbot must have successfully solved their problem.
But this line of thinking has a major blindspot: it doesn’t actually account for resolution.
A customer might leave the conversation without having their issue resolved for a variety of reasons, such as if something important comes up that they have to attend to right away. But even more likely is a scenario where they leave the conversation because the chatbot failed to solve their problem. In this case, containment rate is not only a poor indicator of success, but one that’s actually deeply misleading.
If a chatbot makes a customer repeat themselves multiple times, routes them through various menu options until they reach a dead end, or surfaces information that is unhelpful or irrelevant, it’s easy to see why some customers might feel so frustrated that they give up and close the conversation. Containment rate as a metric, however, would count this interaction as a success.
Challa’s aforementioned Gartner report on chatbot metrics further highlights this blind spot. In one section, he breaks down various chatbot metrics and categorizes them by what they actually measure. While he lists some metrics — like escalation rate and goal completion rate — as measuring “effectiveness,” he lists “containment rate” as measuring just that, “containment.”
Weizhe Yuan, a researcher at New York University whose work focuses on evaluating natural language processing (NLP) tasks, said that selecting appropriate metrics is one of the golden rules of evaluating the success of increasingly-complex NLP-based technologies like chatbots.
“Previously, NLP techniques were focused on traditional NLP tasks such as text categorization, named entity recognition, etc., which usually had some easily measurable metrics. Now, however, NLP techniques are increasingly being applied to open-domain tasks that range from writing novels to rewriting financial documents.”
She added that as companies look to deploy technologies like chatbots for a wider range of uses, it's important to understand what they can and can't do, while also measuring the potential harm they could cause in new use cases.
For years, Kane Simms, a conversational AI and chatbot consultant who works with companies on their customer service strategies, has been shouting from the rooftops that containment rate is the wrong metric for chatbots.
“The containment rate doesn’t tell you anything about whether your customer got what they needed from you. It tells you nothing about how well you’re serving them and how effective your automated channels are,” he wrote in a 2021 blog post , a message he’s since repeated in webinars, LinkedIn posts, and beyond. “It’s also language that makes it sound like your customers are an inconvenient virus that need to be stopped dead in their tracks and isolated in your channels.”
Not to suggest it’s all because of his outspokenness on the topic, but many companies in the space have been drawing the same conclusion (Ada included). Several have started speaking out about the issues with containment rate and even declared they’re moving away from it as a metric, showing the change is gaining momentum across the customer service industry.
In a recent blog post, chatbot company Simplr called containment rate a “red flag metric” that can even be “downright deceptive.” The company says its data shows that up to 20% of “contained tickets” are instances where the customer dropped off because the chatbot was not helpful, rather than because it solved the customer’s problem.
“The metric inflates bot performance and, worse, exposes the extent of customer frustration on a site,” the blog continues.
LivePerson has also made the case that the industry needs to shift its perspective of key metrics in order to better measure chatbot performance.
“We need to assess chatbot success properly, but that doesn’t always mean leaning on familiar key performance indicators,” reads a blog post from the company, which goes on to argue that containment rate doesn’t account for resolution.
Skit.aI has similarly called out containment rate as a problematic north star metric and argues that if the focus is on increasing it, this will actually end up damaging the customer experience.
“Customers may be ending the calls because the Automated Speech Recognition (ASR) engine is not recognizing their voice or words. It can also be that the conversation flows are not optimized, leading to customer frustration,” reads a blog post from the company. “There are several other situations where customer queries are not resolved and causing them to hang up. Here, the containment rate may rise, but at the cost of CX.”
ChatBot, another company in the space, maintains that containment rate is significant, but argues that it’s essential to balance it out with other metrics that will provide a fuller picture, such as user satisfaction and resolution time.
This speaks to the fact that containment rate leaves too much up in the air, and also touches on what Yuan said is one of the most important factors for successfully evaluating NLP-based technologies like chatbots: using multiple metrics.
“In many cases, it's beneficial to use multiple evaluation metrics to get a comprehensive view of your model's performance,” she said. “Different metrics may highlight different aspects of performance.”
With containment rate starting to fade in prominence, it’s clear the industry is entering a new era for chatbot KPIs. And it’s certainly a good time to reevaluate; thanks to advancements in AI (and especially generative AI), the chatbots of today are far ahead of those from just a few years ago, with even more room for improvement for the chatbots of tomorrow.
Customer satisfaction scores (CSAT), for example, are one metric some companies are increasingly leaning on. Unlike containment rate which specifically rewards when customers issues aren’t escalated to a human, CSAT actually targets resolution.
CSAT does have its drawbacks, however. This metric has historically been calculated using data from customer surveys, typically prompted right after the interaction. But critics argue this is a flawed metric and doesn’t paint a comprehensive picture. Many customers choose not to participate in these surveys, and when they do, the questions and methods for answering often lack the nuance needed to truly understand how customers feel. Future advances in how customers share their satisfaction could improve the value of CSAT, but many customer experience leaders believe it currently lacks the rigor necessary to be a central metric.
Citing the foundational shift to an AI-first approach to customer service, Ada is targeting Automated Resolution Rate (AR%) as a new north star KPI for chatbots.
Automated Resolutions are conversations between a customer and a company that are safe, accurate, relevant, and don’t involve a human.
In a blog post discussing the metric, Arnon Mazza, a staff machine learning scientist at Ada, defines automated resolutions as “conversations between a customer and a company that are safe, accurate, relevant, and don't involve a human.” Rather than relying on a customer to provide honest insight after the fact, AR depends uses AI to run the conversation through a safety filter, accuracy filter, and relevancy filter in order to determine if the customer’s inquiry was automatically resolved.
For the accuracy filter, for example, Mazza describes how this is determined by three steps: limiting the knowledge source that the AI distills information from to only the company’s first-party support documentation; running an Elasticsearch index to search for and retrieve the most relevant documents that could contain the most accurate information; and then using several methods — including running a BERT-style natural language inference model on each of the documents and the generated answer — to determine the probability that what was generated is “logically entailed” from the first-party support documents.
With this approach, companies could set thresholds to dictate how confident the AI needs to be to serve an answer, even making it possible to separate the thresholds for relevance and accuracy. They can set higher thresholds for more sensitive questions, or lower ones for topics that may warrant a lower risk tolerance, according to Mazza.
In addition to selecting the right metrics and considering multiple metrics together, Yuan also cited using fine-grained analysis that breaks data into parts as a golden rule of measuring NLP tasks like chatbots. AI has been known to have a “black box” issue, referring to the fact that often no one knows how any specific AI system actually arrives at its outputs (its creators included). Seeking to understand chatbot outputs in more granular ways can not only help understand performance, but also what’s going right, what’s going wrong, and why.
“Instead of looking at the overall performance of the model on the whole dataset, break down the dataset into multiple subsets and see how the model performs in different subsets,” she said. “This will better reveal the strengths and weaknesses of the model.”
Our experts put together this guide with all the info you need about tracking and measuring customer service success in an AI-first organization.
Get the report