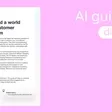
How to build a world-class AI customer service team
Templates and guidance on building a customer service team that uses both AI and human agents to their fullest potential.
Learn MoreFor most businesses, AI adoption is at the top of the to-do list. Enterprises are looking for a trustworthy AI agent for customer service, with over 60% of business owners believing that AI will improve customer relationships and increase productivity.
Those are great numbers, but one hesitation we see often with AI adoption is trust. Can the company trust that the AI won’t go rogue and say something offensive and misrepresent the company? Or that it won’t give false information and hurt their brand’s reputation?
We’ve all seen the horror stories of AI hallucinations across the Internet. But as experts in AI customer service and machine learning, it’s our job to show organizations how Ada’s AI customer service automation platform stands up against their hesitations.
To help businesses better understand what causes hallucinations — and how to deploy a more trustworthy and reliable AI agent — we recently hosted a webinar with OpenAI on this subject.
If you’re considering adopting AI into your organization but the issue of AI hallucinations is holding you back, this is your opportunity to learn from the experts. Here’s a short summary of what we covered — you’ll need to watch the full webinar to get the full scoop:
Check out a snippet from the conversation between myself, a Principal Solutions Consultant at Ada, Ankur Rostogi, Applied AI Scientist at OpenAI Yochai Konig, Ada’s VP of Machine Learning below or watch the full webinar on-demand .
Ankur: I think the two categories that we often talk about are factuality and faithfulness. Factuality might be: how well does the model know a set of known facts? Cases of this are things like birth years, who won a sports game, that kind of thing.
But there's another interesting category of faithfulness, which is, if you give the model some new information, how well does it sort of adhere to that information? Let's say you made up a story about somebody on a different planet, and you had a bunch of details. How well would the model adhere to those?
As far as why these things happen, there's a lot of possible reasons, and we won't be able to talk about all the nitty, gritty details today. But I think some of these are data challenges. It's incumbent on OpenAI to improve the quality of our data set and the data that we train with.
On faithfulness, this is more like an instruction following a challenge. Part of the issue here is how well are the models able to adhere to whatever instructions you provide around wanting it to use certain details you provide at the time. You're making a request. That's like a one or two minute overview. I think those two categories are a helpful way to frame up the problem and then talk about more details later on.
Yochai: It's basically a one context kind of general foundation that the models are built for, for a wide, wide array of tasks — like anything under the sun. And the question is: do we, as practitioners building an application, provide the right context for our domain, for our company?
If we don't, the LLMs or the foundation model will give a general answer that might be wrong for a specific context. It's on us to provide a specific context, so when an end user asks a company-specific question about their pricing policy, the information will come from the company and not from the competitor or other general material that the foundation model was trained on.
Ankur: On the factuality side, oftentimes this is the challenge of a) data, and b) also making sure the models are aware of what they should consider as factual information. I think one thing OpenAI is going to do consistently is try to get the best data we can to make sure the models have the most information available to them. Part of that is increasing the quality of data we have.
There’s also a good mention in the chat about how recently available information is accessible to the models. Today, the models have data and information about the world up to a certain point in time, and there’s a gap between that point in time and the world. We’ll continue to try to bring that gap down.
There are other things as well. We have a lot of amazing users, and there are more mechanisms now for us to capture feedback from those users to better understand if what they see in the model responses is reasonable or not. I think there are ways we can use the information from some of these users to improve the quality of the model responses.
There are some domains — you might say, math and physics — where verifying a correct answer is actually a lot more straightforward than more challenging and more fluid topics like social sciences or something like that. So there’s work that we’ll continue to do around that — for the questions where we can verify more cleanly doing that.
And then on the faithfulness side, there’s a lot of work being done internally to improve the quality of instruction following. One running joke is that these models are the worst that models will ever be. And hopefully that’s always true, right? I’ve also gotten a lot better at instruction and that will continue to happen as well.
For the second half of your question on working with organizations and enterprises, and this extends to our users too, I think a huge component of this is that our visibility into these challenges is often only as good as what we hear from folks out in the world. We have amazing researchers and they’re seeing problems from our side, but hearing from organizations about the kinds of challenges they’re seeing — and the more concrete examples we see — the better understanding gets around where the deficiencies are.
Yochai: We definitely invest a lot on this. I'll equate it to the way that we (humans) solve problems and divide it into four stages.
A lot of the knowledge in the world was built for other humans to read, meaning the author expects the human to apply the human intelligence extract. What we're doing is something similar: taking the knowledge and optimizing it for an AI agent to utilize and to provide accurate answers.
If there’s company knowledge, it’s more superior than the knowledge embedded within the LLM and the foundation model because it’s relevant and more context-specific. We instruct the model to rely on this knowledge as much as possible, following that with human instructions, coaching, and guidance about how to go about solving the problem.
There’s a lot about chain of thinking. Gather the information, extract the relevant information, build the answer.
This is something I developed in my later years. It’s a filter that's saying, should we give this answer right now? Is it the right thing to say? Is it an accurate answer that actually solves the customer issue?
We need to show the answer is relevant, and if it isn’t, what corrective measures can be taken? Ask the user a clarifying question, do a different search to get better information and so forth.
The most important stuff that we, as humans, can do is get feedback. For example, how my performance now in the webinar can be improved. I’ll ask people after it, and I’ll try to adapt for the next opportunity.
Similarly, we're trying to get the human AI manager within the company to give feedback via coaching to the AI — next time you see a similar issue, do it this way, or solve it the other way. Getting the right feedback and coaching from the human to the AI in the right moment, in the right context.
These are the four stages that we're investing a lot in, and we'll keep working to keep improving the accuracy and trustability of the AI agent.
Ankur: I think the first thing is probably just familiarize yourself with all the tools that we give developers. There’s a lot of really interesting, powerful things we provide that developers aren’t always aware of. A couple good examples are the fine tuning customization offerings to get the models to adhere to a certain style, consistency, tone, or area of information. Another is the moderation API.
But there are also other things you need to do too. One thing I would say that’s still underrated even though we know more about it now, is just having a very high quality suite of evals or evaluations.
For better or for worse, the space is moving very quickly. Things are still quite empirical. And even if you have what you think is a well-built system ahead of time, it doesn’t mean much until you’re able to guarantee that it’s working the way you expect it to. We usually recommend that organizations take some time to put together a set of expected answers. And when you’re iterating on these systems to consider the results you’re seeing on those evals over time. In the same way that automated tests are a critical part of building software at scale, evals are probably the analogy for building AI systems at scale.
Yochai: The mindset is continuous improvement. Think about it like an employee that you onboard. They are intelligent, they know a lot, but they’re not used to the context of the company, the practices, the policy, and the new products coming. You need to keep training and guiding. What does this mean in practice?
There’s a lot of stuff that can happen, and the question is, how to be alerted quickly. And then as employees, how you can guide your agent to deal with this as quickly as possible. Minds of iterative continuous improvement is the most important ingredient, along with the right tooling and measurement to actually execute.
What causes hallucinations, and are there steps you can take to deploy a more trustworthy and reliable AI agent? Watch the full webinar with OpenAI to find out.
Watch the webinar